When it comes to keeping equipment in top shape, traditional maintenance approaches just don’t cut it anymore. Reactive maintenance leads to unexpected downtime, and even scheduled preventive maintenance sometimes results in unnecessary part replacements. That’s where predictive maintenance steps in — using real-time data to forecast when equipment will fail, so maintenance happens only when it’s truly needed.
But predictive maintenance can’t function effectively without one key ingredient: data — and lots of it. More importantly, it needs to be the right kind of data, collected automatically, consistently, and in real time. That’s where automated data collection plays a crucial role.
In this blog, let’s break down how automated data collection powers predictive maintenance strategies, improves efficiency, reduces costs, and ensures reliability across industrial environments.
What is Automated Data Collection?
Automated data collection is the process of capturing, processing, and storing equipment performance data without human intervention. It typically involves the use of:
- Sensors (temperature, vibration, pressure, humidity, etc.)
- IoT (Internet of Things) devices
- Machine controllers and PLCs
- Data loggers
- Edge computing systems
These systems gather data directly from assets and feed it into a centralized system — usually a CMMS (Computerized Maintenance Management System) or an IIoT platform — where it can be analyzed for trends and patterns.
Why Predictive Maintenance Relies on Automated Data Collection
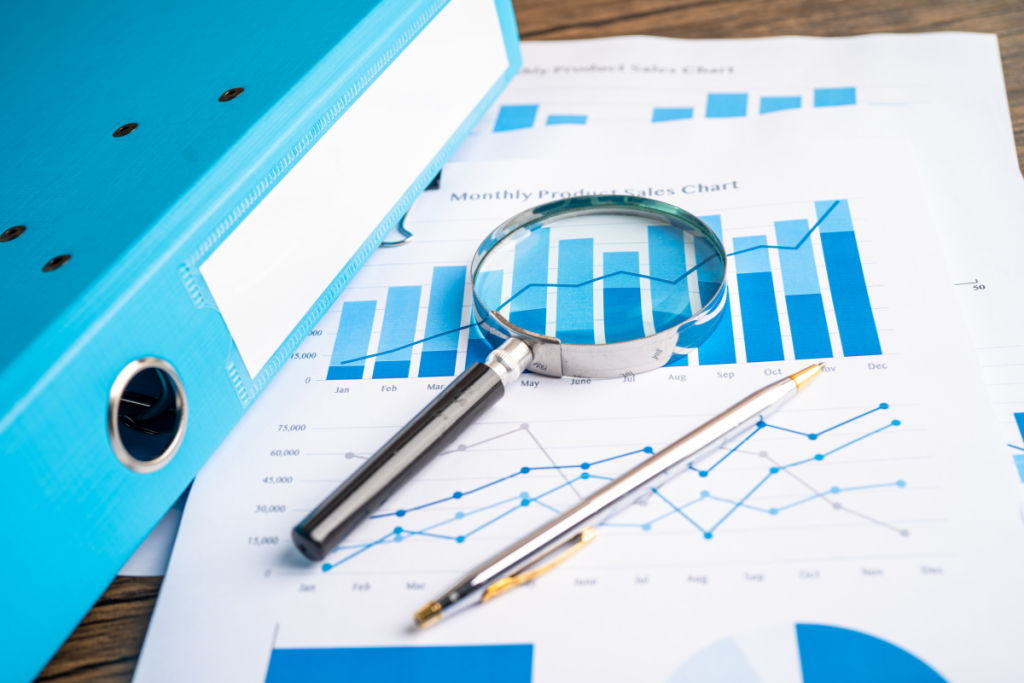
Predictive maintenance depends on real-time insights derived from operational data. This level of precision and responsiveness can only be achieved through automated data collection systems. Below are eight key reasons why automated data collection is fundamental to the success of predictive maintenance strategies:
1. Enables Continuous Monitoring
Automated data collection allows machines and equipment to be monitored continuously, capturing performance data 24/7 without manual intervention. This uninterrupted flow of data is crucial for spotting gradual changes or anomalies that indicate early signs of wear or potential failure.
Without continuous monitoring, subtle yet critical deviations in equipment behavior may go unnoticed. Automated systems eliminate blind spots and provide maintenance teams with a complete picture of asset health at all times.
2. Supports Multi-Parameter Tracking
Industrial assets often rely on multiple operating parameters—like vibration, temperature, pressure, and voltage—to function efficiently. Automated data collection enables all these variables to be tracked simultaneously through sensors and integrated monitoring systems.
By capturing multiple data points together, organizations can better understand the interdependencies between different components and identify complex failure patterns that would be missed through isolated checks.
3. Improves Data Accuracy and Reliability
Manual data entry is prone to human error, inconsistencies, and time delays, all of which can compromise the reliability of predictive insights. Automated data systems eliminate these issues by ensuring that data is captured precisely and consistently, in real time.
High-quality data enhances the accuracy of predictive algorithms and minimizes false alerts. It also improves the decision-making process by providing trusted data that engineers and analysts can confidently act upon.
4. Enables Real-Time Alerts and Quick Response
Automated systems can instantly detect when an asset operates outside of normal thresholds and generate real-time alerts. These alerts enable maintenance teams to intervene quickly—before minor issues turn into major failures.
Real-time response is key to minimizing unplanned downtime and extending equipment life. With automated triggers in place, organizations can act immediately on critical data, reducing both repair costs and operational risks.
5. Feeds Predictive Analytics and AI Models
Predictive maintenance relies on historical and live data to fuel AI, machine learning, and statistical models that forecast failures. Automated data collection ensures a continuous feed of structured, clean, and timestamped data for these models to learn and evolve.
Without this data pipeline, predictive models become ineffective or outdated. Automated collection ensures models are always trained on the latest conditions, improving their accuracy and relevance over time.
6. Allows Scalability Across Assets and Sites
As organizations grow, the number of assets and volume of data increases exponentially. Automated data collection provides the scalability required to monitor hundreds or thousands of machines across multiple sites without increasing manual workload.
With centralized dashboards and cloud integration, decision-makers can manage asset health across geographies in real time. This scalability makes predictive maintenance not just feasible but sustainable for large-scale operations.
7. Enables Historical Trend Analysis
Long-term data collected automatically forms a rich database of historical performance trends. These trends help maintenance teams identify recurring issues, evaluate the effectiveness of interventions, and forecast future maintenance needs more accurately.
Such historical insights are crucial for optimizing maintenance schedules, planning parts inventory, and improving equipment design over time. Manual data collection simply cannot match the depth or reliability of such insights.
8. Integrates Seamlessly with CMMS and IoT Ecosystems
Automated data collection systems can be integrated with Computerized Maintenance Management Systems (CMMS), IoT platforms, and cloud-based analytics tools. This integration streamlines the entire maintenance workflow—from detection to resolution.
When sensor data directly triggers work orders or preventive tasks in a CMMS, it reduces administrative overhead and shortens the maintenance cycle. The result is a more proactive, efficient, and connected maintenance ecosystem.
Technologies Enabling Automated Data Collection
Here are the main technologies that make automated data collection possible in a predictive maintenance ecosystem:
IoT Sensors
These are the frontline tools for collecting real-time performance data like temperature, vibration, humidity, voltage, etc.
Edge Devices
Edge computing allows initial data processing to happen close to the equipment, reducing latency and bandwidth load on centralized systems.
Cloud Integration
Automated data can be pushed to cloud-based platforms for long-term storage, advanced analytics, and dashboard visualization.
AI and Machine Learning
Machine learning algorithms analyze massive data sets from multiple sources to detect patterns that humans can’t, enabling smarter maintenance predictions.
CMMS & EAM Systems
Modern CMMS and EAM platforms ingest real-time data to update asset health, generate work orders, and track performance KPIs automatically.
Benefits of Automated Data Collection in Predictive Maintenance
In industrial settings, predictive maintenance is only as effective as the data that feeds it. Automated data collection provides the scale, speed, and precision necessary to monitor complex machinery, anticipate failures, and optimize asset performance. From high-frequency sensor input to seamless system integrations, this approach delivers measurable advantages across engineering, operations, and maintenance functions.
Here are the key technical benefits it offers:
1. High-Frequency Data Sampling for Micro-Deviation Detection
Automated systems collect data in sub-second intervals, capturing minute fluctuations that are often invisible to manual inspections. This high-resolution input enables the detection of micro-deviations in operational behavior, Ncritical in identifying early-stage mechanical or electrical anomalies before they escalate.
2. Multivariate Signal Correlation for Root Cause Isolation
Advanced data collection setups monitor multiple parameters simultaneously, such as vibration, pressure, temperature, and flow. Correlating these signals in real-time helps isolate root causes faster, especially in systems where interdependent component failures are common, improving diagnostic precision.
3. Condition-Based Triggering of Maintenance Workflows
Automated data pipelines can directly initiate maintenance actions based on real-time thresholds or trend analysis. This enables condition-based scheduling, where service is triggered by asset behavior rather than calendar cycles, leading to more efficient labor allocation and fewer unnecessary interventions.
4. Lifecycle Load Profiling for Asset Degradation Modeling
Continuous data capture provides a detailed load profile of equipment usage over time. This helps in modeling how specific stressors—like overloading, high duty cycles, or environmental fluctuations—affect degradation rates, supporting better lifecycle forecasting and capital planning.
5. Sensor-Driven Elimination of Human Data Latency and Bias
Manual reporting introduces delays, inconsistencies, and subjectivity into the data pool. Sensor-driven automation removes these risks by delivering real-time, objective input directly from the asset to the analytics layer, improving the quality of machine learning models and predictive insights.
6. Closed-Loop Integration with CMMS and Industrial IoT Platforms
Automated data systems can feed CMMS platforms with live condition data, enabling dynamic creation of work orders, spare parts requisitions, and failure logs. This closed-loop integration bridges operations and maintenance, enhancing responsiveness and reducing downtime.
7. Anomaly Pattern Recognition Using Historical Sensor Archives
Historical sensor data enables the identification of recurring anomaly patterns across identical or similar assets. Over time, this builds a reference library that improves fault classification accuracy and supports predictive modeling based on real-world failure signatures.
8. Scalable Edge-to-Cloud Data Architecture for Enterprise-Wide Monitoring
Automated collection solutions can operate at the edge for low-latency analysis while synchronizing data to the cloud for centralized visibility. This hybrid architecture allows organizations to monitor thousands of distributed assets, standardize predictive strategies, and enforce consistent reliability practices.
Implementing Automated Data Collection in Your Predictive Maintenance Strategy
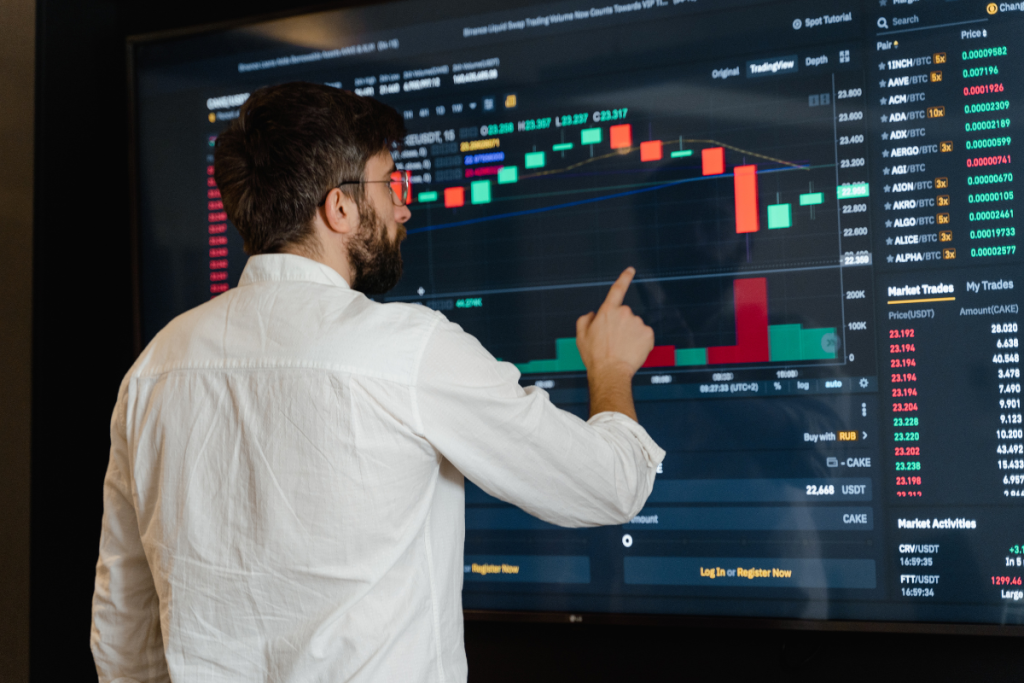
To leverage the full potential of predictive maintenance, automated data collection must be planned, integrated, and optimized systematically. It’s not just about installing sensors but establishing a comprehensive data infrastructure that captures, transmits, processes, and applies information in a meaningful way. Below is a step-by-step process that outlines how organizations can effectively implement automated data collection within their maintenance strategy:
Step 1: Identify Critical Assets and Parameters
Begin by conducting an asset criticality analysis to identify which machines or components are most vital to your operations and carry the highest risk or cost of failure. Subsequently, once prioritized, define the parameters that reflect their health—such as temperature, vibration, pressure, RPM, noise levels, lubrication quality, etc. Ultimately, this ensures data collection focuses on indicators that are truly predictive of asset performance.
Step 2: Select Appropriate Sensors and Instrumentation
Choose sensors that are compatible with both the asset type and the required data parameters. Consider sensor type (e.g., piezoelectric for vibration, RTD for temperature, ultrasonic for leak detection), data resolution, communication protocols, and environmental conditions. Ensure that sensors support real-time or near-real-time data transmission and can be integrated with your existing systems.
Step 3: Establish Connectivity Infrastructure
Deploy communication protocols and network layers that can reliably handle the volume and frequency of sensor data. Depending on your environment, this may include a combination of wired (Ethernet, RS485) and wireless (Wi-Fi, Zigbee, LoRaWAN, NB-IoT) networks. Edge devices or industrial gateways should be implemented to aggregate sensor data and perform basic preprocessing if required.
Step 4: Integrate with a Centralized Data Platform
Connect all data sources to a centralized platform capable of handling large-scale time-series data—such as an IIoT platform, data lake, or cloud-based CMMS. This platform will serve as the foundation for further analytics and automation. Use APIs or standard protocols like MQTT, OPC-UA, or Modbus TCP to ensure seamless integration across equipment types and vendors.
Step 5: Implement Real-Time Monitoring and Alerting
Set threshold values, rules, or condition-based logic that enables the system to flag anomalies or potential failures as they occur. Create real-time dashboards to visualize the data, and set up automated alerts through SMS, email, or system notifications to inform maintenance personnel of critical issues that require immediate action.
Step 6: Enable Data Analytics and Machine Learning
Use historical and real-time data to build predictive models using statistical analysis or machine learning techniques. These models can detect degradation patterns, forecast remaining useful life (RUL), and provide actionable insights. Use platforms that provide built-in predictive analytics capabilities if internal data science expertise is limited.
Step 7: Automate CMMS and Workflow Integration
Connect the data analytics layer with your CMMS to automatically generate work orders based on condition triggers or predicted failure events. This integration ensures that maintenance actions are both timely and aligned with actual equipment needs, enabling a seamless transition from detection to resolution.
Step 8: Establish Feedback Loops and Continuous Improvement
Continuously monitor the system’s performance and refine thresholds, sensor placement, and predictive models based on observed outcomes. Incorporate feedback from technicians to improve model accuracy and response logic. This creates a dynamic maintenance environment that evolves with asset behavior and organizational priorities.
Step 9: Ensure Data Security and Compliance
As industrial data becomes a valuable asset, implement encryption protocols, access controls, and secure communication channels to protect data integrity and prevent cyber threats. Ensure compliance with industry standards such as ISO/IEC 27001 or NIST guidelines for cybersecurity in industrial systems.
Step 10: Scale Across Sites and Asset Types
Once validated at a pilot level, subsequently, extend the system across additional facilities, production lines, or asset classes. In addition, use scalable architectures like edge-to-cloud platforms and modular sensor kits to streamline deployment. Furthermore, ensure enterprise-wide consistency in data standards and integration practices to maintain quality and comparability of data insights.
Summing it up
Implementing automated data collection transforms how businesses approach predictive maintenance, turning data into actionable insights that drive better decisions. It helps organizations stay ahead of potential failures, optimize asset performance, and reduce downtime. With real-time visibility and more accurate forecasting, companies can maximize operational efficiency and minimize unnecessary costs. Ready to take your maintenance strategy to the next level? Reach out to us at contact@terotam.com to learn how we can help.