Asset Health Reliability Insight (AHRI) refers to the structured process of continuously gathering, analyzing, and interpreting asset-related data to assess current condition, predict future failures, and guide reliability-centered maintenance strategies. It combines operational technology (OT), information technology (IT), and engineering practices to deliver actionable intelligence about asset integrity, degradation rates, and remaining useful life (RUL).
Rather than relying on reactive or calendar-based maintenance, AHRI enables a shift toward predictive and prescriptive maintenance models. It focuses on detecting early-stage anomalies, understanding root causes, and reducing mean time between failures (MTBF) while improving mean time to repair (MTTR).
Why is the AHRI Important for Reliability Engineering?
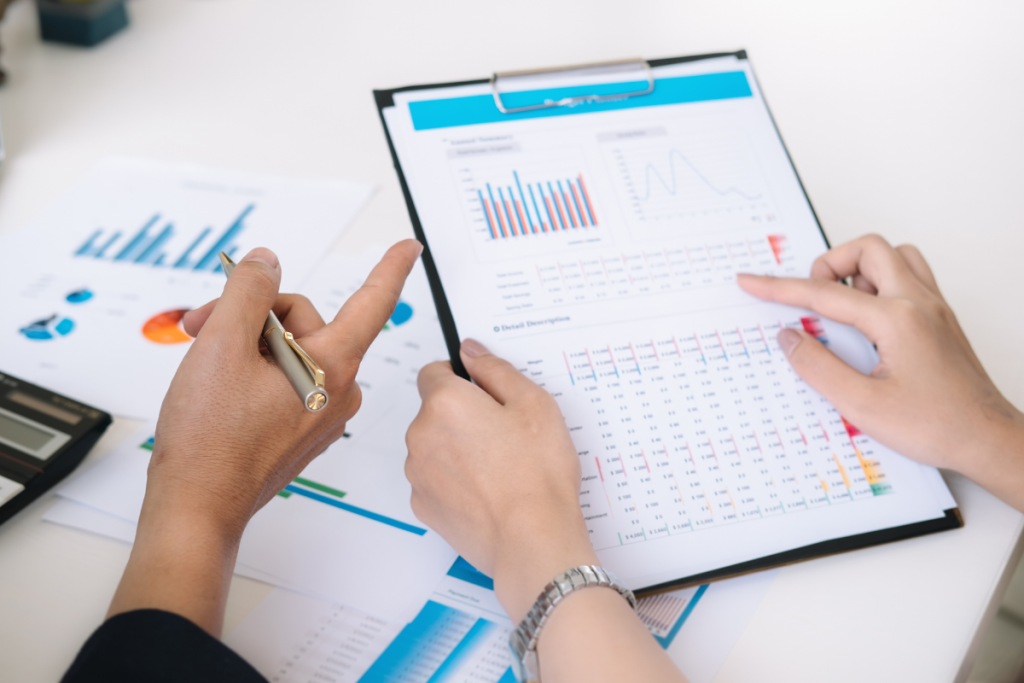
In asset-intensive industries, equipment reliability directly impacts production throughput, operational safety, and maintenance budgets. Unplanned failures not only lead to downtime but often result in cascading effects across interconnected systems.
Asset Health Reliability Insight plays a key role in minimizing:
- Unexpected failure modes in rotating and static equipment.
- Safety risks arising from undetected equipment fatigue or wear.
- Maintenance inefficiencies from over- or under-servicing.
- Capital waste by avoiding premature replacements.
With the help of having condition awareness and failure prediction into reliability workflows, organizations can achieve higher equipment availability (A), improved OEE (Overall Equipment Effectiveness), and lower total cost of ownership (TCO).
6 Core Components of AHRI Systems
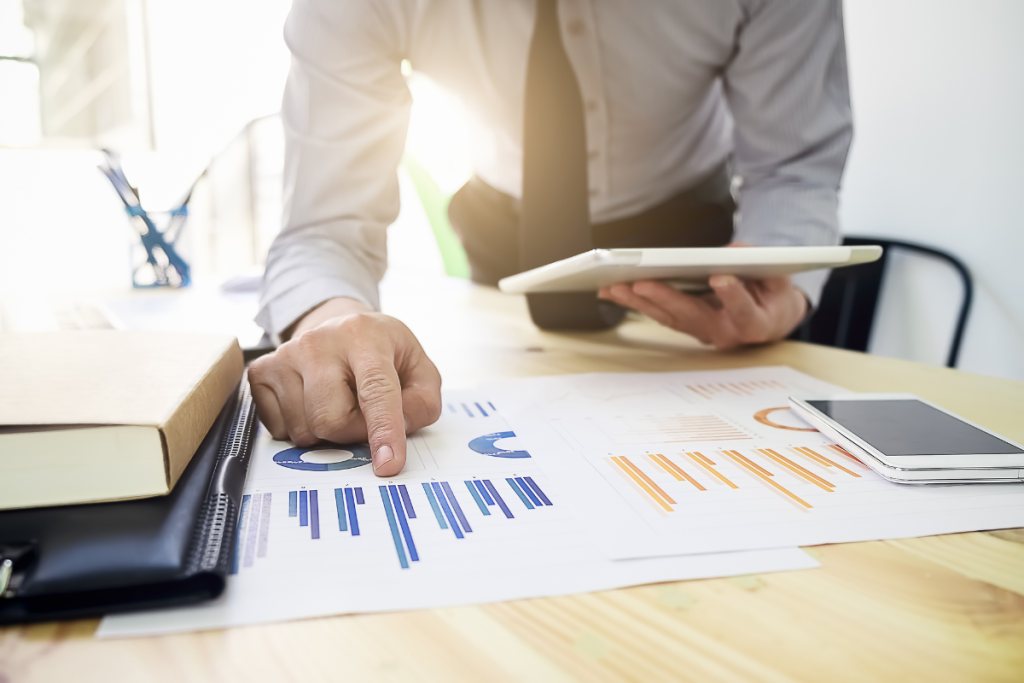
An effective AHRI framework integrates multiple engineering disciplines and digital systems. Below are the core building blocks:
1. Sensor-based data acquisition
Sensors (accelerometers, strain gauges, thermocouples, pressure transducers, etc.) collect real-time physical and operational parameters. These inputs feed into edge devices or PLCs for preprocessing.
Key metrics captured:
- Vibration (velocity, acceleration, FFT)
- Temperature gradients
- Pressure differentials
- Acoustic emissions
- Oil condition and contamination
- Electrical currents and harmonics
2. Condition-based asset modeling
These models evaluate how an asset performs under current conditions. Thresholds are defined using OEM specs or empirical data. Deviation from baseline values triggers alerts or work orders.
Examples:
- Bearing temperature exceeding 90°C
- Vibration spectrum showing imbalance or misalignment
- Oil viscosity below ISO 32 standard
3. Reliability-centered data analytics
Reliability engineers use structured data (run hours, fault logs) and unstructured data (technician notes, visual inspections) to identify degradation paths and prioritize failure modes.
Tools used:
- Failure Modes and Effects Analysis (FMEA)
- Root Cause Failure Analysis (RCFA)
- Fault Tree Analysis (FTA)
- Reliability Block Diagrams (RBDs)
4. Historical trend analysis
Data historians and CMMS integrations help analyze asset performance over months or years. Trends in performance decline, repair intervals, and failure types reveal actionable patterns.
Insights derived:
- MTBF vs. asset age
- Correlation between ambient humidity and insulation breakdown
- Early indicators of cavitation in pumps based on NPSH readings
5. Predictive analytics and RUL estimation
Machine learning models trained on historical failure events forecast RUL. Techniques like regression modeling, anomaly detection, and neural networks help refine maintenance timelines.
Common models used:
- Weibull distribution analysis
- Cox Proportional Hazards model
- LSTM (Long Short-Term Memory) for time-series prediction
6. Risk-based asset prioritization
Each asset is ranked based on failure impact, likelihood, and criticality. This ensures that limited resources are directed to high-risk or production-critical assets.
Assessment factors:
- Financial loss per hour of downtime
- Impact on safety or regulatory compliance
- Redundancy availability
What are the Top Technologies enabling AHRI?
Implementing asset health insights requires integration across multiple platforms:
- CMMS (Computerized Maintenance Management System): Tracks work orders, service logs, and spare parts.
- EAM (Enterprise Asset Management): Manages asset hierarchies, lifecycles, and cost histories.
- Condition Monitoring Systems: Real-time acquisition and diagnostics from vibration, oil, and thermographic data.
- AI/ML Platforms: Enable advanced pattern recognition, clustering, and prediction of complex failure mechanisms.
- IoT Gateways and Edge Devices: Process and normalize data at the source before sending to cloud analytics engines.
- Digital Twin Models: Provide simulation-based testing of operational scenarios without impacting live systems.
Applicability of AHRI System – Use cases across industries
Asset Health Reliability Insight is being actively implemented across several sectors where asset failure can lead to operational disruptions, safety risks, or financial losses. The specific challenges may vary across industries, but the underlying goal remains the same: to maximize the lifespan and performance of critical equipment while reducing reactive interventions.
Manufacturing Industry
In manufacturing environments, downtime affects not just output but entire supply chains. With AHRI, factories can monitor real-time performance metrics on production lines, identify when machines are trending toward failure, and initiate corrective action before the issue becomes costly.
Predictive insights are used on CNC machines, conveyor systems, and industrial robots to reduce scrap rates and unplanned stoppages.
Energy and Utilities Industry
For power plants, substations, and water treatment facilities, uninterrupted service is non-negotiable. AHRI supports utilities by offering early warnings on transformer overheating, partial discharges in switchgear, and degradation of rotating turbines.
This helps teams prioritize inspections and optimize grid reliability without waiting for visible faults or forced shutdowns.
Oil & Gas Industry
This industry faces extreme operating conditions and tight safety regulations. AHRI systems are used to monitor offshore and onshore assets, including compressors, pumps, and pipelines. With continuous tracking of vibration signatures and flow anomalies, asset reliability teams can detect cavitation, seal failures, and early signs of corrosion or fatigue before they compromise throughput or safety.
Rail and Transport Industry
Railway operators use AHRI to improve rolling stock reliability and reduce service disruptions. Onboard sensors monitor wheel health, axle stress, brake system wear, and suspension performance.
Combined with route-specific usage data, this insight supports dynamic maintenance schedules, reducing both costs and downtime while improving commuter safety.
Key Outcomes and KPIs Gained from Asset Health Reliability Insights
Implementing AHRI systems goes beyond improving individual maintenance activities. It reshapes how organizations think about reliability, lifecycle cost, and resource allocation. By moving from time-based to condition-based and predictive models, companies achieve more control over asset performance, with measurable benefits across the board.
Mean Time Between Failures (MTBF)
Tracking MTBF helps quantify the reliability of individual components or systems. As AHRI identifies failure precursors earlier, maintenance can be scheduled more effectively, resulting in increased MTBF and more consistent uptime.
Mean Time to Repair (MTTR)
With access to accurate failure diagnostics and early alerts, maintenance teams can respond faster and with the right tools or parts in hand. This reduces repair duration and restores assets to operation quicker.
Planned vs. Unplanned Maintenance Ratio
AHRI drives a higher ratio of planned maintenance events by forecasting failures before they happen. This not only avoids last-minute interventions but also allows for better coordination between production and maintenance schedules.
Asset Availability Ratio
Real-time health insights reduce both sudden failures and unnecessary maintenance. As a result, critical assets spend more time operating and less time out of service, pushing availability percentages closer to optimal targets.
Maintenance Cost per Unit of Output
By reducing over-maintenance and preventing expensive breakdowns, AHRI contributes directly to lowering maintenance expenditure relative to production volume or operating hours.
Remaining Useful Life (RUL) Accuracy
Using machine learning and historical trend analysis, AHRI systems refine RUL predictions over time. Improved accuracy means maintenance actions are performed neither too early (wasting resources) nor too late (risking failure), enhancing overall planning and lifecycle cost efficiency.
Wrapping it up
Equipment doesn’t fail without warning—it shows signs. The challenge is being able to catch those signs early enough to act on them. That’s exactly what Asset Health Reliability Insight is built for. By tapping into real-time data, historical trends, and predictive analytics, you can move away from guesswork and toward well-informed decisions that keep your operations running efficiently.
If you’re exploring smarter ways to manage your assets and reduce downtime, we’re here to help.
Get in touch at contact@terotam.com to see how AHRI can fit into your maintenance strategy.